Ethical Considerations of Generative BI: Balancing Innovation with Responsibility
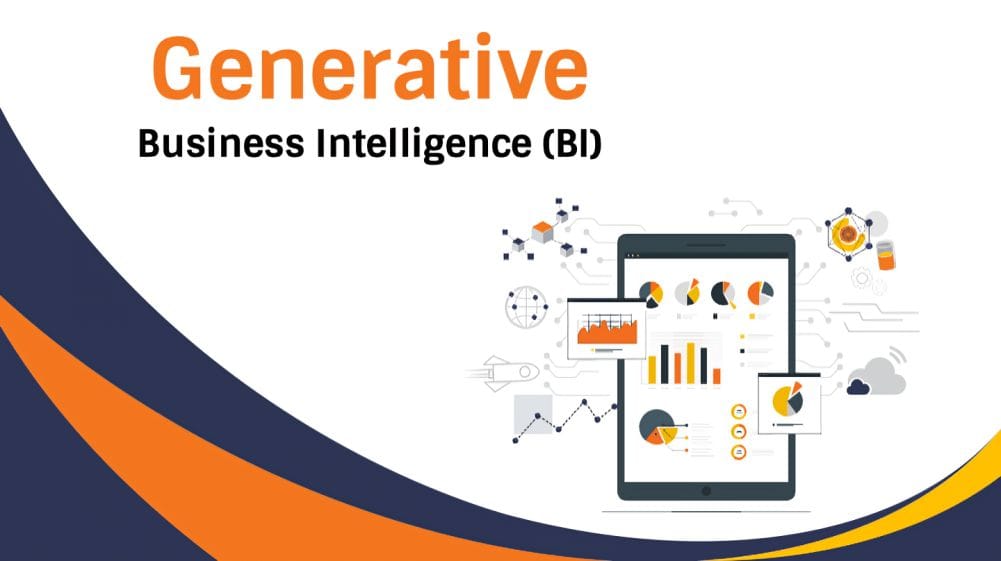
As information technology rapidly changes in this world, every business is looking forward to utilizing the power of Generative Business Intelligence to make better decisions and optimize operations for innovation. Being a bleeding-edge technology, Generative BI will alter industries with predictive models, including data creation and automation of complex tasks. Yet inseparable from innovation is the requirement that consideration should be given to ethical concerns. Like any other potent tool, how can we take due care that it is being used responsibly, weighing the exuberance of innovation with the caution of ethics?
What is Generative BI?
Generative BI is a specialized branch of business intelligence, applying machine learning and AI to generate new data, models, or insights. Conversely from traditional BI systems, which analyse past data in search of patterns and trend analysis, Generative BI produces outputs that will contribute to forecasting future trends, simulating scenarios, and even automating decisions. This makes it a potential enabler in such industries as health, finance, and retail, as the sector will more and more need predictive analytics for growth and efficiency of operations.
In finance, this intelligence might forecast the prices of stocks or build portfolio recommendations by analysing market data. It models patient data in healthcare to project the outcomes of treatment or to generate personalized care plans. While such applications reveal a vast use of Generative BI, they also bring up vital ethical questions.
Ethics Becoming an Asset in Technology
As companies adopt Generative BI, they must also face the ethical challenges that come with using such powerful technology. Ethics in technologies go well beyond mere compliance with regulations; it includes a broader understanding of societal impacts AI will bring, and making sure innovations are put to use for collective good. This has become all the more critical since AI and technologies of machine learning spread so rapidly across industries. Ethics should be considered as the moral compass for IT solutions, where uncomfortable questions are considered:
Are we making sure the system is fair?
– Are we safeguarding user privacy?
– Is our system transparent and an accountable one?
Being upfront regarding these issues will help avoid some of the unintended consequences and will ensure that Generative BI is leveraged responsibly.
Key Ethical Concerns in Generative BI
Though Generative BI offers a number of advantages, it also raises quite a few ethical concerns. Some of these are:
- Bias and Discrimination: Most of the outcomes will be unjust or prejudicial if the generative models are built on prejudiced data.
- Privacy Concerns: Typically, Generative BI requires large volumes of data. Such sensitive information is sometimes part of it. How do we protect the privacy of individuals while leveraging such data?
- Transparency and Accountability: The generative BI models are seen as “black boxes” without any notion of how the decisions come about and might thus have accountability.
- Security Risks: Every AI-powered system is vulnerable in one way or another to data breach and security threats. Generative BI needs security to be an important feature at the front to prevent misuse.
Let’s consider each of these in more detailed ways: –
Bias and Discrimination in Generative BI
The biggest ethical risk in Generative BI is related to bias. These systems generate models with the help of historical data, and this could further capture certain biases in that data. For example, when a Generative BI model is trained on biased hiring data, even the act of picking job candidates for a job position may unconsciously lead it to favour a particular demographic over others.
All these risks can be mitigated only if the companies invest in creating diverse, unbiased datasets and regularly auditing their models for fairness. Only then will techniques like fairness-aware algorithms and bias detection tools make sure that the output of Generative BI is equitable and non-discriminatory.
Privacy Issues in Generative BI
Generative BI is driven by data. The more diversified data are available, the better it can make predictions and provide insights. On the other side, this may raise some substantial privacy issues. For instance, if a healthcare organization tries to model patient data using Generative BI, there is a chance that sensitive medical information may be disclosed or misused.
Companies can only address such a concern by applying rigorous data protection practices with encryption, anonymizing the data, and following the best practice of privacy regulations such as the GDPR. In addition, methods that guarantee differential privacy-anonymize the data in a manner that insights can still be had-could protect personal privacy yet enable innovation.
Accountability and Transparency
Generative BI models are often considered to be opaque or “black boxes,” since it is impossible, if not hard, to understand exactly how decisions are arrived at. The lack of transparency can be a problem-perhaps especially so in industries such as finance or healthcare, where decisions have great real-world consequences.
This means that making transparent these different models and decision-making mechanisms that go with Generative BI systems to the developers and the end-users. For instance, a company should document its model training, data used to train such models, and provide explanations for the output provided. Besides, accountability frameworks need to be in place to build trust in using Generative BI systems.
Security Risks in Generative BI
Like any technology dealing in extensive form with data, generative BI systems have a risk of security. A leakage or cyber-attack would eventually result in the loss of sensitive business or personal information. Financial losses or reputational losses would, as a consequence, emerge after that. If tampered with in any way, the generative model might originate results that are false or deceptive in nature. This would cause substantial harm.
This would reduce security risks if the companies implemented some cybersecurity best practices, such as encryption, multi-factor authentication, and regular security audits. Added to this are AI-specific security measures, adversarial training in models could make them resistant to manipulation.
Balancing Innovation with Ethical Responsibility
While its potential is huge, the obligation of companies must be to balance innovation with ethical responsibility. This means embedding ethics in software development from data collection to model deployment. By doing so, companies can ensure that their innovations are groundbreaking yet in tune with the values of society.
Notice how some businesses lead the way in responsible AI, embedding ethical directives and best practices into their generative BI systems. They know full well that trust will have to be earned with their customers, regulators, and the general public if long-term success is to be had.
Regulatory and Legal Considerations The regulatory landscape will equally change as Generative BI continues to develop. Governments and international organizations are just beginning to draft new regulations that focus on the ethical dilemmas created by AI and BI systems. Current regulations, such as the GDPR, provide a framework for protection and privacy, but more specific regulations concerning AI will most likely be developed.
This means that organizations should drive the development by adhering to the existing regulations and those which may be issued for enforcement in the future. This could also mean taking best practices on ethical AI, performing impact assessments, rating transparency, and accountability in Generative BI systems.
Ethics and the Future of Generative BI
In general, generative BI is bright, but at the same time, it is going to be defined by impending ethics. As technology keeps upping the ante, issues will be thrown up: everything from fairness in AI-generated decisions to security of increasingly complex systems.
Being proactive about ethics would lower major risks and position a company leading the market in responsible innovation. It requires continuous dialogue, various stakeholder collaboration, and commitment to the development of AI for societal benefit.
Conclusion
But with great power comes great responsibility, and Generative BI is no different. Addressing the ethical considerations-bias, privacy, transparency, security-companies can tap into Generative BI’s full potential with confidence that it will be used responsibly. In a world of changing technology, balancing innovation with ethical responsibility is not just best practice; it’s necessity.
FAQs
-
- What would be the major ethical issue in Generative BI?
Issues in Generative BI almost refer to probable biases and discriminations during modeling, along with privacy issues related to this large volume of data. - How does one make sure that the use of Generative BI in companies is ethical?
Fairness, transparency, and accountability are necessary in ensuring ethical usage by embedding it into a company’s development process, auditing models for bias, and protecting user privacy. - What is the significance of regulations with respect to ethical Generative BI?
Although regulations like GDPR create a framework that can guide data protection and privacy, we are likely to see more articulated AI-oriented regulations as Generative BI is developed and expanded further. - Does innovation play an important role in cases involving strict ethical policies?
Yes, innovation may flourish where guidelines for ethics can be instituted. In this case, ethics build trust with users, create accountability, and help to evade possible problems before they occur. - What is the future of ethics in Generative BI?
The future of ethics in Generative BI concerns emerging challenges: those of bias, transparency, and security. These are constantly updated with regulatory developments on best practice.
- What would be the major ethical issue in Generative BI?